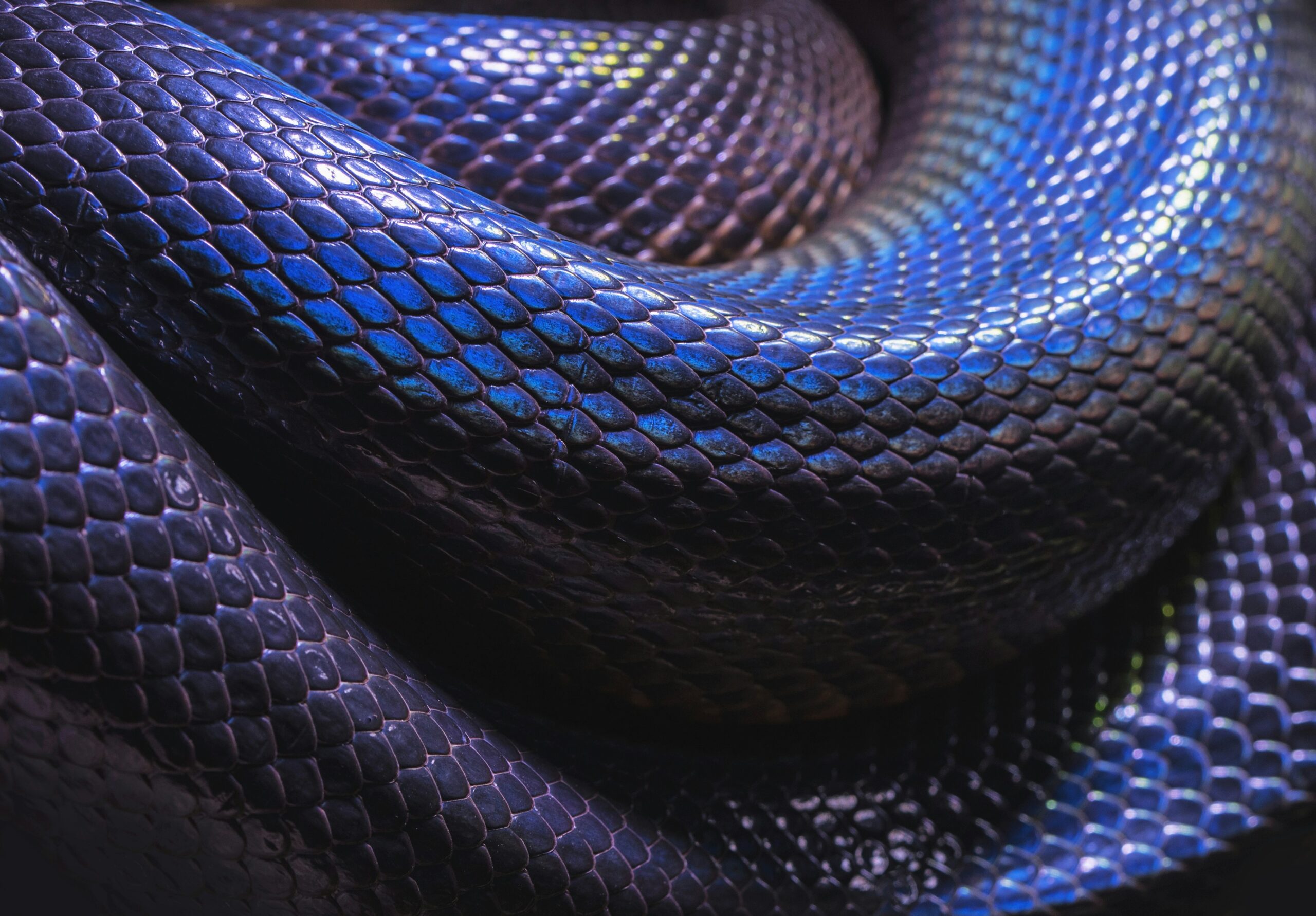
Tim O’Loughlin, Lecturer in Government, Flinders University
POLICY PERSPECTIVES #10, February 2024 | DOWNLOAD THIS POLICY PERSPECTIVE |DOI.ORG/10.25957/xg6f-ka98
Governments worldwide habitually deploy data analytics tools to streamline the task of governing, ideally leading to more effective, efficient, and economical processes. Governments’ use of data analytics tools can be clustered into three categories: descriptive analytics (e.g. identifying voting irregularities, detecting welfare fraud); prescriptive analytics (e.g optimising use of public transport resources, pooling information from multiple public agencies to assist emergency responses); and predictive analytics (e.g. forecasting post-earthquake tsunamis, identifying spatial areas with elevated risk of suicides).
Inevitably, problems have arisen with governments’ use of data analytics, mostly caused by political agendas and public sector management failings. But the frequency and gravity of the problems seem to peak when two conditions are present: (1) when analytic tools are used to predict; and (2) when those predictions are used by governments to support the exercise of their legitimate coercive powers.
Take, for example, predictive policing. One of the pioneers in the e use of predictive policing was the Santa Cruz Police Department in the State of California in 2016. The tool relies on the statistical evidence that crime is concentrated into specific areas in a city. If your house is robbed, the probabilities of you and your neighbours being robbed again are much higher. Combining that data with historic arrest data for the area makes it possible to identify not only where crime is more likely to occur but who is more likely to be committing the crime. This allows the police to call in the “likely” suspects; tell them they have been identified; offer some help; and, most of all, warn them that now they have been counselled, they will throw the book at them and their associates if they go on to commit a crime. For five consecutive years, the tool was selected for the GovTech 100 list in the US. Six months after that Santa Cruz dropped it along with several other cities such as Los Angeles, New Orleans and Chicago. The reason? –The murder of George Floyd in 2020 elevated scrutiny of systemic bias against African Americans by police forces around the country. The tool came under criticism for basing predictions on historic data from patrols and arrests concentrated disproportionately in black neighbourhoods.
The use of data analytics for child protection has also been fraught with challenges.The algorithm for forecasting the extent of risk of maltreatment of young children was originally developed in 2012 by a team at the University of Auckland. The idea was to supplement clinical assessments with a tool which would allow for a “cost-effective method of targeting early prevention services”. Using historic data of children in contact with New Zealand’s public benefit system, the research findings included that 48% of those rated in the top decile of risk had suffered maltreatment by 5 years of age.
The tool suffered an early demise in New Zealand when then Minister Anne Tolley reacted to a recommendation from officials for an observational two-year trial which would allow “sufficient follow-up time to assess whether children identified by the PM (predictive model) as at high risk of an adverse outcome(s) did in fact suffer that outcome”. The Minister understood that to mean that officials were proposing to suspend services to test the veracity of the tool. Her response, “not on my watch, these are children, not lab rats”, spelt the end. And, in Scotland, a similar exercise suffered the same fate.
However, the Auckland team subsequently tendered successfully for provision of such a tool for the Allegheny County, Pennsylvania. Its use there has not been without controversy, particularly as a result of Virginia Eubanks excellent case study of its application in the field. However, it survived and spawned variants which are being used by many other jurisdictions in the US as well as several cities in Europe.
It is interesting to contemplate what these differing outcomes say about the impacts of political culture. Was the failure in New Zealand merely the result of using a clumsy form of words or does its rejection there and acceptance in the US suggest more important differences? Certainly, the Allegheny team made much of the “careful implementation” process applied there but there may be other factors at work.
For instance, US culture seems more embracing and less suspicious of technological innovation generally and government use of technology specifically. As an example, the light-handed regulation for protecting privacy in the US may be compared with Europe’s comprehensive and forceful General Data Protection Regulation.
A second factor might be that these innovations often hold out the promise of cost efficiencies. This may be more compelling for US city and regional governments which typically are under-resourced by developed world standards.
Finally, these tools may be better suited for use in highly individualist cultures like the US. Emily Keddell has argued that the discourse around the use of the tool to protect “vulnerable children” is “refracted through a neoliberal responsibilisation agenda aimed at their parents”, treating them as solely responsible for their circumstances thereby exempting government from responsibility. By way of contrast, others have argued that the use of just one variable – poverty – would achieve around the same predictive accuracy as the 136 used by the Allegheny County algorithm.
The same neo-liberal flavour is to be found in predictive policing. The underlying belief is that individuals can be held solely accountable for their behaviours without consideration of various circumstances which may impel them to offend.
The danger is that predictive tools may be used imperceptibly by governments to convert their social responsibilities into individual ones. The deficits in accountability and transparency usually associated with these tools make resisting that process particularly challenging for democratic practice and for public sector management.
Further reading
Eaglin, J.M. (2017) Constructing Recidivism Risk. Articles by Maurer Faculty. 2647. Available from: https://www.repository.law.indiana.edu/facpub/2647
Eubanks, V. (2018) Automating Inequality: How High-Tech Tools Profile, Police and Punish the Poor. New York, NY: St Martin’s Press.
Keddell, E. (2015) The ethics of predictive risk modelling in the Aotearoa/New Zealand child welfare context: Child abuse prevention or neo-liberal tool? Critical Social Policy, 35(1), 69–88. https://doi.org/10.1177/02610 18314543224
Mr Tim O’Loughlin
Tim is a Lecturer in Government at Flinders University.
With a rich and varied career spanning politics, the commercial sector, and public service, Tim has honed his expertise in areas such as public sector financial management, public administration, and energy policy. From serving as chief-of-staff to Australia’s Minister for Foreign Affairs to leading departments and commissions in South Australian Government, Tim has dedicated himself to driving impactful change. Over the past 12 years, Tim has shared his knowledge as a lecturer in public policy schools. His passion lies in fostering innovation and facilitating collaboration between the public and private sectors to address complex challenges.